FRTE 1:N Identification
These comments provided by Neurotechnology are based on NIST FRTE 1:N Identification ongoing evaluation results reviewed on November 28, 2023.
NIST FRTE 1:N Identification tests the suitability of biometric facial recognition algorithms for civil and law enforcement applications with two sets of experiments. The "Identification" set of experiments covers civilian use by setting criteria to minimize errors and assist in automated decisions. The "Investigation" set of experiments aims for law enforcement use by using the evaluation parameters to produce results for further human review to validate or dismiss returned candidates. Both sets of experiments include the the same scenarios and datasets.
See FRTE official page for more information.
The Face Recognition Technology Evaluation (FRTE) started in July, 2023, as a successor of the previous FRVT Ongoing evaluation of face verification algorithms. The FRTE 1:N Identification track allows vendors to submit their algorithms once every three months.
Neurotechnology algorithm has been ranked in the top 4% of most accurate results matching frontal and profile mugshots scenarios among 452 submissions by 128 different vendors. The algorithm showed top results among border control supervised (Visa vs Border, Border vs Border ΔT ≥ 10 YRS) and unsupervised (Visa vs Kiosk) scenarios. Also, considering the template size, the algorithm showed the best results among all other submissions with the same template size.
Eight scenarios with six datasets were tested. Two experiments were performed in each scenario:
- Identification – intended to test civilian use cases. This experiment used a threshold set to limit the False Positive Identification Rate (FPIR) to 0.3%. FPIR is the proportion of non-mated searches producing one or more candidates above threshold. The False Negative Identification Rates (FNIR) is the proportion of mated searches failing to return the mate above threshold. The use of thresholding supports use of face recognition in making mostly automated decisions e.g. for access into facility.
- Investigation – intended to test law enforcement use cases. This experiment used a threshold set to zero FPIR, and the algorithm should return a fixed number of candidates. FNIR is the proportion of mated searches for which the algorithm does not place the correct candidate at rank 1. The use of face recognition without a threshold supports investigating uses where it is assumed and necessary that a human expert will have to review the candidates returned from each search. For mated-searches the human is tasked with finding the correct mate; for non-mated searches the reviewer must reject all the candidates.
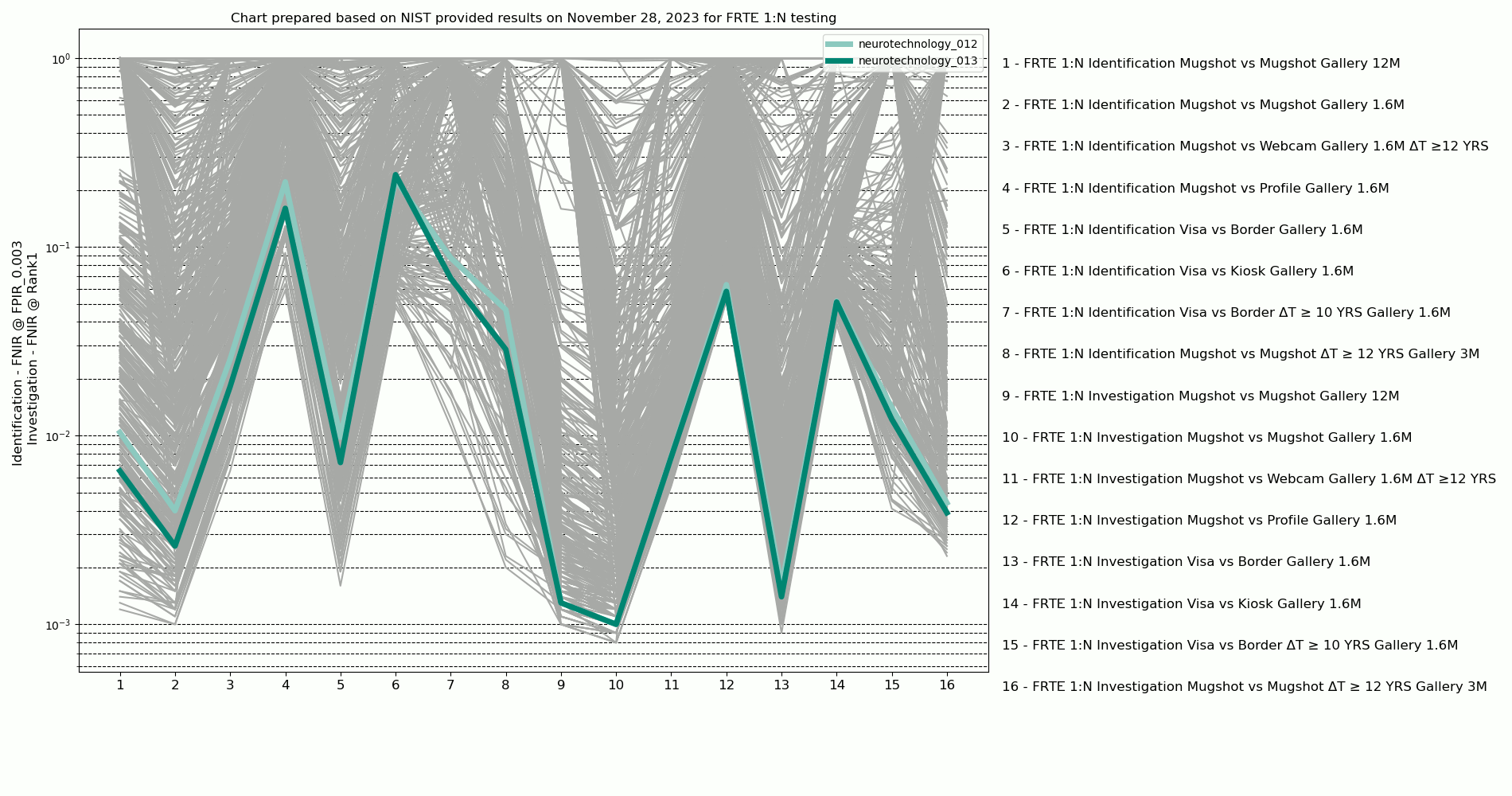
The horizontal axis on the chart corresponds to the scenarios and experiments:
Identification experiments
- Mugshot vs Mugshot, 12 million gallery
- Mugshot vs Mugshot, 1.6 million gallery
- Mugshot vs Webcam, 1.6 million gallery
- Mugshot vs Profile, 1.6 million gallery
- Visa vs Border, 1.6 million gallery
- Visa vs Kiosk, 1.6 million gallery
- Border vs Border ΔT ≥ 10 years, 1.6 million gallery
- Mugshot vs Mughsot ΔT ≥ 12 years, 3 million gallery
Investigation experiments
- Mugshot vs Mugshot, 12 million gallery
- Mugshot vs Mugshot, 1.6 million gallery
- Mugshot vs Webcam, 1.6 million gallery
- Mugshot vs Profile, 1.6 million gallery
- Visa vs Border, 1.6 million gallery
- Visa vs Kiosk, 1.6 million gallery
- Border vs Border ΔT ≥ 10 years, 1.6 million gallery
- Mugshot vs Mughsot ΔT ≥ 12 years, 3 million gallery
Mugshot vs Mugshot, 12 million gallery
Identification – Neurotechnology algorithm accuracy in this experiment was 0.65% FNIR at 0.3% FPIR. The most accurate contender showed 0.12% FNIR at the same FPIR.
Investigation – Neurotechnology algorithm accuracy in this experiment was 0.13% FNIR at Rank1. The most accurate contender showed 0.10% FNIR at Rank1.
Scenario overview
- 12 million images from the Mugshot dataset were used for persons' enrollment.
- In part of the cases more than one image corresponded a unique person.
- Each face template was compared against all other enrolled templates.
- The comparisons were fully zero-effort, meaning there was no pre-grouping by gender, age or other covariates.
Mugshot vs Mugshot, 1.6 million gallery
Identification – Neurotechnology algorithm accuracy in this experiment was 0.26% FNIR at 0.3% FPIR. The most accurate contender showed 0.10% FNIR at the same FPIR.
Investigation – Neurotechnology algorithm accuracy in this experiment was 0.10% FNIR at Rank1. The most accurate contender showed 0.08% FNIR at Rank1.
Scenario overview
- 1.6 million images from the Mugshot dataset were used for persons' enrollment.
- In part of the cases more than one image corresponded a unique person.
- Each face template was compared against all other enrolled templates.
- The comparisons were fully zero-effort, meaning there was no pre-grouping by gender, age or other covariates.
Mugshot vs Webcam, 1.6 million gallery
Identification – Neurotechnology algorithm accuracy in this experiment was 1.82% FNIR at 0.3% FPIR. The most accurate contender showed 0.65% FNIR at the same FPIR.
Investigation – Neurotechnology algorithm accuracy in this experiment was 0.77% FNIR at Rank1. The most accurate contender showed 0.54% FNIR at Rank1.
Scenario overview
- Images from Mugshot and Webcam datasets were used for persons' enrollment. About 86% of the images were from the Mugshot dataset.
- 1.6 million images from the Mugshot dataset were used.
- In part of the cases more than one image corresponded a unique person.
- Each enrolled face template from the Webcam dataset was compared against all enrolled templates from the Mugshot dataset.
- The comparisons were fully zero-effort, meaning there was no pre-grouping by gender, age or other covariates.
Mugshot vs Profile, 1.6 million gallery
Identification – Neurotechnology algorithm accuracy in this experiment was 16.00% FNIR at 0.3% FPIR. The most accurate contender showed 5.89% FNIR at the same FPIR.
Investigation – Neurotechnology algorithm accuracy in this experiment was 5.81% FNIR at Rank1. The most accurate contender showed 5.17% FNIR at Rank1.
Scenario overview
- Images from Mugshot and Profile datasets were used for persons' enrollment. About 86% of the images were from the Mugshot dataset.
- 1.6 million images from the Mugshot dataset were used.
- In part of the cases more than one image corresponded a unique person.
- Each enrolled face template from the Profile dataset was compared against all enrolled templates from the Mugshot dataset.
- The comparisons were fully zero-effort, meaning there was no pre-grouping by gender, age or other covariates.
Visa vs Border, 1.6 million gallery
Identification – Neurotechnology algorithm accuracy in this experiment was 0.72% FNIR at 0.3% FPIR. The most accurate contender showed 0.16% FNIR at the same FPIR.
Investigation – Neurotechnology algorithm accuracy in this experiment was 0.14% FNIR at Rank1. The most accurate contender showed 0.09% FNIR at Rank1.
Scenario overview
- Images from Visa and Border datasets were used for persons' enrollment.
- 1.6 million images from the Border dataset were used.
- In part of the cases more than one image corresponded a unique person.
- Each enrolled face template from the Border dataset was compared against all enrolled templates from the Visa dataset.
- The comparisons were fully zero-effort, meaning there was no pre-grouping by gender, age or other covariates.
Visa vs Kiosk, 1.6 million gallery
Identification – Neurotechnology algorithm accuracy in this experiment was 24.12% FNIR at 0.3% FPIR. The most accurate contender showed 4.77% FNIR at the same FPIR.
Investigation – Neurotechnology algorithm accuracy in this experiment was 5.10% FNIR at Rank1. The most accurate contender showed 3.87% FNIR at Rank1.
Scenario overview
- Images from Visa and Kiosk datasets were used for persons' enrollment.
- 1.6 million images from the Visa dataset were used.
- In part of the cases more than one image corresponded a unique person.
- Each enrolled face template from the Kiosk dataset was compared against all enrolled templates from the Visa dataset.
- The comparisons were fully zero-effort, meaning there was no pre-grouping by gender, age or other covariates.
Border vs Border with 10+ years difference, 1.6 million gallery
Identification – Neurotechnology algorithm accuracy in this experiment was 6.82% FNIR at 0.3% FPIR. The most accurate contender showed 1.23% FNIR at the same FPIR.
Investigation – Neurotechnology algorithm accuracy in this experiment was 1.22% FNIR at Rank1. The most accurate contender showed 0.45% FNIR at Rank1.
Scenario overview
- 1.6 million images from the Border dataset were used for persons' enrollment.
- In part of the cases more than one image corresponded a unique person.
- Age attribute was used to exclude template pairs, which represented same or different persons with age difference of less than 10 years at the capture moment, from the comparison.
- Each face template was compared against all other enrolled templates.
Mugshot vs Mughsot with 12+ years difference, 3 million gallery
Identification – Neurotechnology algorithm accuracy in this experiment was 2.86% FNIR at 0.3% FPIR. The most accurate contender showed 0.20% FNIR at the same FPIR.
Investigation – Neurotechnology algorithm accuracy in this experiment was 0.39% FNIR at Rank1. The most accurate contender showed 0.23% FNIR at Rank1.
Scenario overview
- 3 million images from the Mugshot dataset were used for persons' enrollment.
- In part of the cases more than one image corresponded a unique person.
- Age attribute was used to exclude template pairs, which represented same or different persons with age difference of less than 12 years at the capture moment, from the comparison.
- Each face template was compared against all other enrolled templates.
Datasets overview
These datasets were used for the experiments.
Mugshot
The Mugshot dataset contains high quality frontal images, which were taken in a controlled environment.
- Subjects' pose is generally excellent with mild pose variations around the frontal.
- Variable image sizes, with many being 480x600 pixels.
- Medium JPEG compression ratio.
Profile
The Profile dataset contains images, which were collected during the same session as the frontal mugshot photograph, in the same standardized photographic setup.
- The images were stored with the same image sizes and JPEG compression ratio as in the Mugshot dataset.
Webcam
The Webcam dataset contains images, which were collected using an inexpensive webcam attached to a flexible operator-directed mount.
- Subjects' pose may be non-frontal with some pitch and yaw variations, associated with the rotational degrees of freedom of the camera mount.
- There are low contrast images due to varying and intense background lights. Also the images have poor spatial resolution due to inexpensive camera optics.
- All images are 240x240 pixels.
- High JPEG compression ratio also affected images' quality.
Visa
The Visa dataset contains images, which were collected in a controlled environment with high-quality equipment.
- Subjects' pose is generally excellent.
- All images are 300x300 pixels.
- Some of the images have medium JPEG compression ratio, while the others have light compression.
Border
The Border dataset contains images, which were collected in a field environment at border crossing points.
- Subjects' pose may be non-frontal with some pitch and yaw variations, associated with the rotational degrees of freedom of the camera mount.
- Part of the images features other subjects visible behind the primary subject.
- The images are often low contrast due to varying and intense background lights. Also the images feature poor spatial resolution due to inexpensive cameras used.
- Most images are 320x240 pixels.
- Medium JPEG compression ratio.
Kiosk
The Kiosk dataset contains images, which were collected from subjects whose attention was focused on interaction with an immigration kiosk. The camera is fixed above a touchscreen display, and the images are captured without checking if the subject's pose is correct.
- Subjects' pose is mostly pitched down, sometimes exceeding 45 degrees. Yaw-angle variation is mild.
- Tall individuals are sometimes cropped due to the kiosk setup. This is often just above the eyes and can occur at the nose or mouth. Conversely, short individuals are sometimes cropped such that only the top part of the face is visible.
- There are some images with other subjects visible behind the primary subject.
- Some images are under-exposed due to visible ceiling lighting in the background.
- The images are 320x240 pixels.